Tricentis launches qTest Copilot to empower QA Organisations to Ship Quality Software Faster
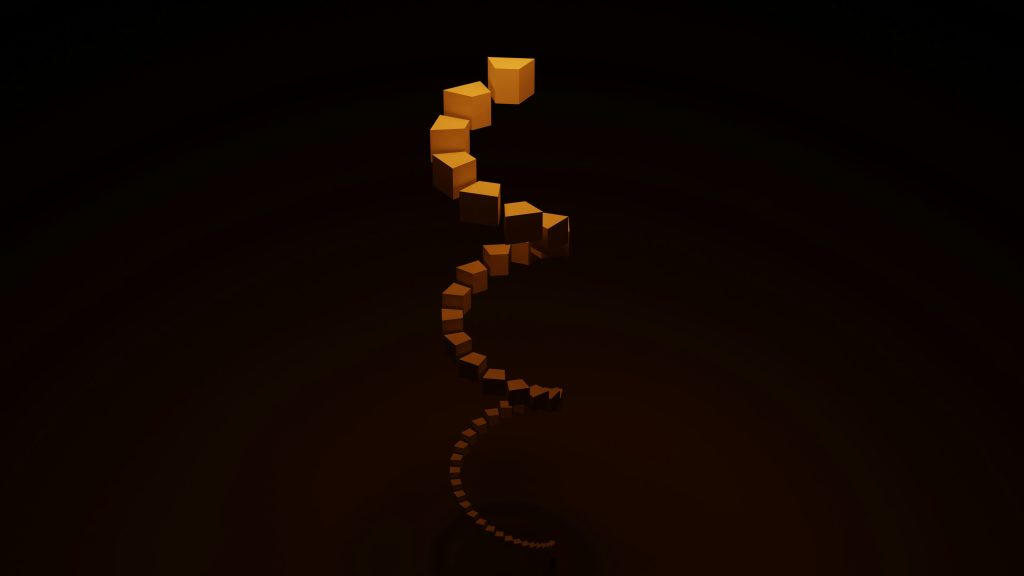
Tricentis, a global leader in continuous testing and quality engineering, today announced the expansion of its test management and analytics platform, TricentisqTest, with the launch of Tricentis qTest Copilot. The latest addition to its suite of generative AI-powered Tricentis Copilot solutions, qTest Copilot harnesses the power of generative AI to simplify and accelerate test case generation, allowing for greater test coverage and higher-quality software releases. qTest Copilot is a generative AI assistant that automatically drafts test cases and test steps based on source documents and user requirements, offering considerable time-saving benefits when compared to manual approaches. Embedded into the newest version of the qTest platform, qTest Copilot combines Tricentis’ scalable and unified test management technology, with new AI-augmented features to allow QA and developer teams to greatly accelerate software delivery. Users can quickly create test coverage of any application, as well as explore unidentified quality gaps by broadening the test scope to include tests for additional scenarios and unexpected events. With a single click, both test steps and expected results are generated in seconds, enabling users to deliver higher-quality releases more confidently and with fewer escaping defects. The addition of generative AI features into qTest also enables more common and consistent test case descriptions, which both new and existing teams can use to create standards for how test cases are written across their entire test coverage. Other features include: Select and easily control which projects and users are enabled for qTest Copilot. Approve drafted test cases after modifying, deleting, or creating new steps as needed. Prompt qTest Copilot to summarize for more concise outputs or to elaborate with more details. Regenerate test steps or the entire test case without losing the overall test scope. Further features will also follow in 2025, including increased functionality for test case discovery, whereby users can map requirements to existing test cases, as well as test case and requirement review, which aims to analyze and improve the quality of existing assets in the qTest environment. “Developer and QA teams today are looking to drive meaningful and measurable improvements to the test coverage of their applications, all while driving significant productivity gains,” comments Mav Turner, Chief Product and Strategy Officer, Tricentis. “Feedback from our beta program suggests that qTest Copilot is enabling users to create complex test cases far more quickly than ever before, while also identifying gaps in test coverage that might have otherwise been overlooked. By automating these critical testing steps, teams can focus their efforts on higher-value activities, ultimately accelerating delivery timelines and improving overall software quality.” Recent Tricentis research found that DevOps practitioners ranked testing as the most valuable (60%) area of AI investment across the software delivery lifecycle, and almost one-third (32%) of respondents estimate AI-augmented DevOps tools will save teams over 40 hours per month—equivalent to an entire workweek. The addition of qTest Copilot to the Tricentis suite of generative AI-powered test automation assistants follows the launch of Tricentis Testim Copilot in April and Tricentis Tosca Copilot in June. Tricentis Copilot solutions utilize generative AI to help enterprises streamline the testing process for faster cycles, more efficient testing, and better business outcomes. qTest Copilot will be included with all new purchases of the latest version of qTest. Existing qTest customers can also begin leveraging the enhanced benefits of qTest Copilot by purchasing an upgrade from their previous version of qTest to the new Tricentis qTest Enterprise AI version. Learn more about how qTest Copilot, Tosca Copilot and Testim Copilot can help QA and development teams move faster and achieve better quality at https://www.tricentis.com/products/copilot Additional Resources: Webinar: Introducing qTest Copilot Blog: Introducing qTest Copilot Press release: Announcing Tricentis Copilot: Accelerating Application Testing Speed and Quality with Generative AI Report: Tricentis AI-Augmented DevOps Report 2024 Blog: Introducing Tricentis Copilot Website: Tricentis Copilot Website: AI-powered quality solutions Webinar: Our vision for the future of AI-driven testing About Tricentis Tricentis is a global leader in continuous testing and quality engineering. The Tricentis AI-based, continuous testing portfolio of products provide a new and fundamentally different way to perform software testing. An approach that’s automated, fully codeless, and intelligently driven by AI. It addresses both agile development and complex enterprise apps, enabling enterprises to accelerate their digital transformation by dramatically increasing software release speed, reducing costs, and improving software quality. Widely credited for reinventing software testing for DevOps, cloud, and enterprise applications, Tricentis has been recognized as a leader by all major industry analysts, including Forrester, Gartner, and IDC. Tricentis has more than 3,000 customers, including the largest brands in the world, such as McKesson, Allianz, Telstra, Dolby, and Vodafone. To learn more, visit https://www.tricentis.com. Get in touch For event sponsorship enquiries, please get in touch with calum.budge@31media.co.uk For media enquiries, please get in touch with vaishnavi.nashte@31media.co.uk
AI decreases human-generated content, limiting data for training AI
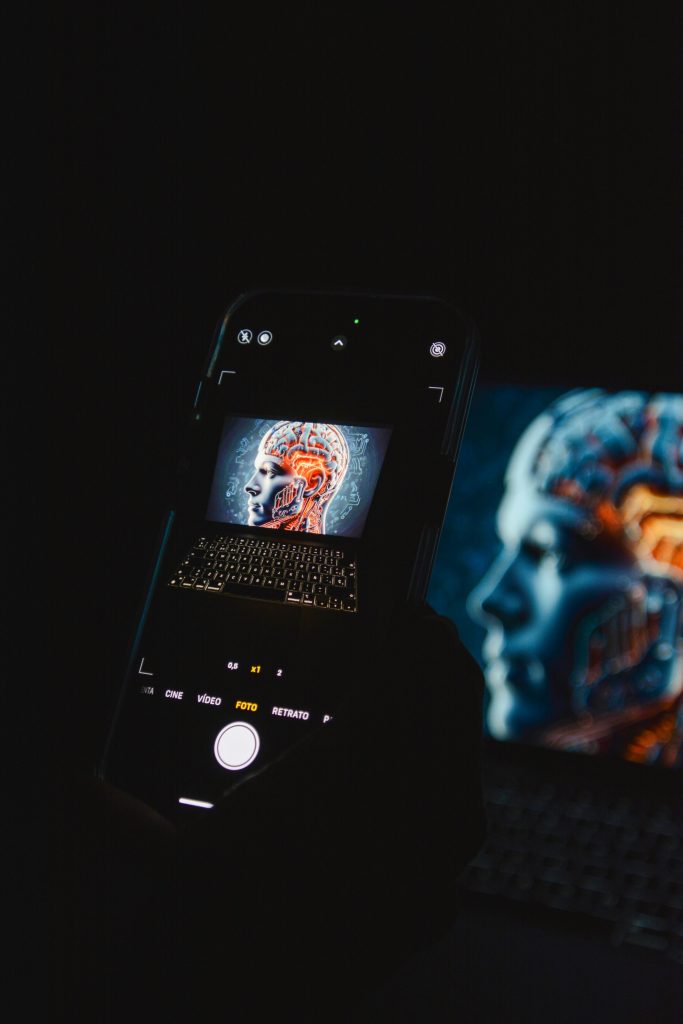
The use of ChatGPT has led to a decrease in human-generated content with people asking and answering fewer questions online, according to new research from Corvinus University of Budapest. Content and discussions online are used by people to learn new things and solve problems, and essential for training AI, particularly Large Language Models (LLMs) like ChatGPT. Johannes Wachs, Associate Professor at Corvinus University, and colleagues from UCL and LMU Munich investigated the impact of ChatGPT on the generation of open data on Stack Overflow, an online Q&A platform for computer programmers and an essential source of training data for LLMs. The researchers found that, after the introduction of ChatGPT, there was a sharp decrease in human content creation: ChatGPT users are less likely to post questions and answers on the platform or visit the platform regularly. As people use ChatGPT more instead of online knowledge databases or platforms which allow discussion, displacing the human behaviour which generates the data it is trained on, the quality and quantity of data available for training future AI decreases. “The decreased production of open data will limit the training of future models. LLM-generated content itself is likely an ineffective substitute for training data generated by humans to train new models. Training an LLM on LLM-generated content is like making a photocopy of a photocopy, providing successively less satisfying results,” says Professor Wachs. The researchers explain that we should prioritise encouraging people to exchange information and knowledge online with each other, and not only rely on AI and LLMs. These findings were first published in the journal PNAS Nexus. Get in touch For event sponsorship enquiries, please get in touch with calum.budge@31media.co.uk For media enquiries, please get in touch with vaishnavi.nashte@31media.co.uk
How open-source is shaping the future of innovation
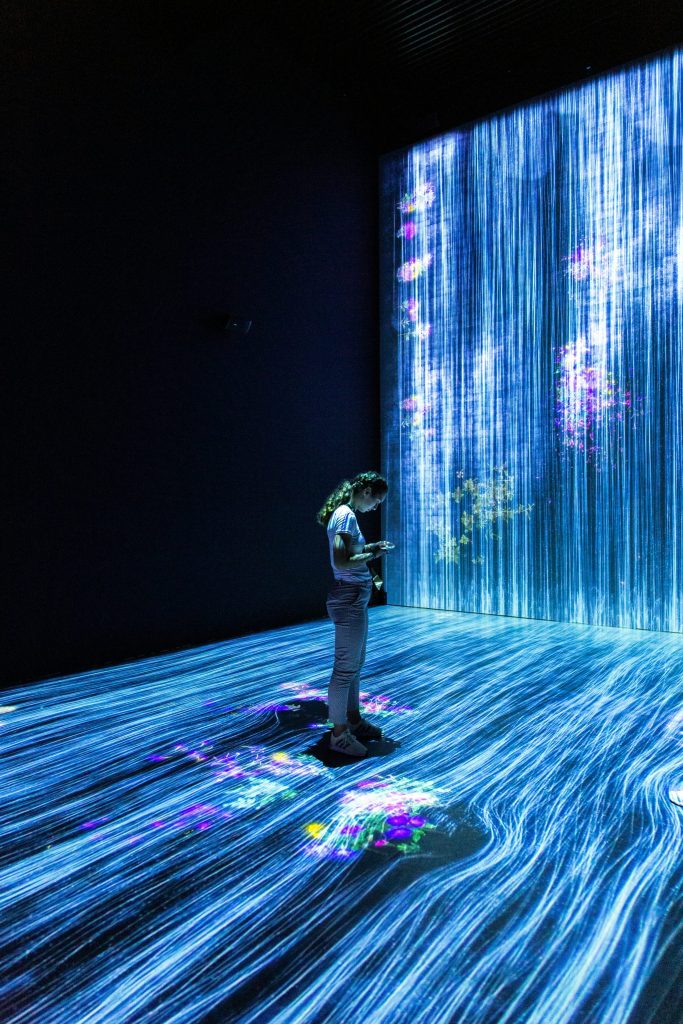
Open-source technology has significantly shaped how industries approach complex technical challenges and foster innovation. It has expanded beyond software to impact hardware and systems design, opening up new pathways for researchers, engineers, and tech enthusiasts to develop advanced solutions. This collective approach fuels innovation and contributes to sustainable development by creating accessible, adaptable tools that solve real-world problems at scale. Democratising open-source technology and science Open-source technology fundamentally changes how we access and utilise high-tech tools. Traditionally, advanced instruments like oscilloscopes, signal generators, and spectrum analysers were accessible primarily to well-funded research labs. With open-source tools such as Red Pitaya’s “pocket lab,” engineers and students can now access powerful measurement and testing capabilities in a compact, cost-effective format. This allows universities, startups, and even independent researchers and enthusiasts to innovate without being restricted by cost or proprietary limitations. Moreover, open-source solutions offer essential benefits such as customisation and adaptability, empowering users to modify or extend tools to meet specific needs. In education, students gain hands-on experience, and in the professional world, industries from aerospace to telecommunications use these tools for testing and development. In practical terms, this accessibility fosters a continuous feedback loop where users refine, improve, and adapt tools in real time. Accelerating innovation through collaboration The open-source model thrives on community collaboration. Rather than isolated development, open source operates on a principle of collective intelligence, where thousands of developers and engineers contribute, review, and enhance each other’s work. This ecosystem allows for faster progress, improved quality, and a richer feature set than proprietary models might achieve. Peer review and open access enable users to build on existing solutions, cutting down on time-to-market and reducing resource expenditure on redundant development. Mateja Lampe Rupnik mentioned one example involving a radiation detection device sent to the International Space Station to stream real-time data back to Earth. Developed by physics students and their professors, this project demonstrates the potential of open source to support ambitious projects in a collaborative, resource-efficient manner. By eliminating entry barriers and encouraging collective problem-solving, open source enables advancements in high-tech fields that might otherwise be constrained. Sustainable solutions for a changing world Sustainability is an increasingly urgent issue, and open-source technology plays a valuable role in addressing it. Traditional corporate-led solutions are often restricted by bureaucracy and profit motives. By contrast, open source allows smaller, agile teams to develop groundbreaking solutions to environmental challenges. Startups have leveraged open-source tools to create technologies for environmental monitoring, air quality analysis, and even animal conservation. For example, Red Pitaya STEMlab has been used in projects to monitor harmful gas emissions, track deforestation in the Amazon, and measure water quality in remote areas. By offering cost-effective, adaptable solutions, open source helps make environmental monitoring accessible, especially in underrepresented regions. In Namibia, for instance, an open-source project uses sensor technology to prevent human-wildlife conflicts without harming animals, ensuring the safety of both people and local wildlife. These types of projects demonstrate the impact of open-source tools in promoting sustainable practices and meeting critical environmental needs. Supporting startups and disruptive innovation Many startups have successfully leveraged open-source technology to accelerate their development cycles and reduce costs. Open-source technology enables startups to create prototypes quickly and access a global community for testing and refinement, making it easier to attract investors and bring viable products to market. Open-source hardware not only supports early-stage companies but also enables them to bring sophisticated solutions to sectors that require high accuracy and reliability, like environmental monitoring and medical technology. By focusing on shared development and reducing overhead, open source creates a clear path for emerging tech companies to innovate sustainably. The future of open source in high-tech innovation Looking forward, open source will continue to be a key driver in areas where adaptability and rapid innovation are essential. Fields like healthcare, education, and environmental technology will likely see the most immediate benefits as open-source models make advanced solutions more accessible and customisable. In medical technology, for instance, the combination of open-source hardware and artificial intelligence could address staff shortages and resource constraints by automating diagnostic processes or supporting remote healthcare in underserved areas. Open source offers a model for sustainable progress, enabling a wide range of individuals and institutions to develop solutions at a global scale. As technology becomes more collaborative and interconnected, open source remains a powerful enabler for creating solutions to complex global challenges, driving innovation, and building a more sustainable future. Get in touch For event sponsorship enquiries, please get in touch with calum.budge@31media.co.uk For media enquiries, please get in touch with vaishnavi.nashte@31media.co.uk
How AI is transforming businesses
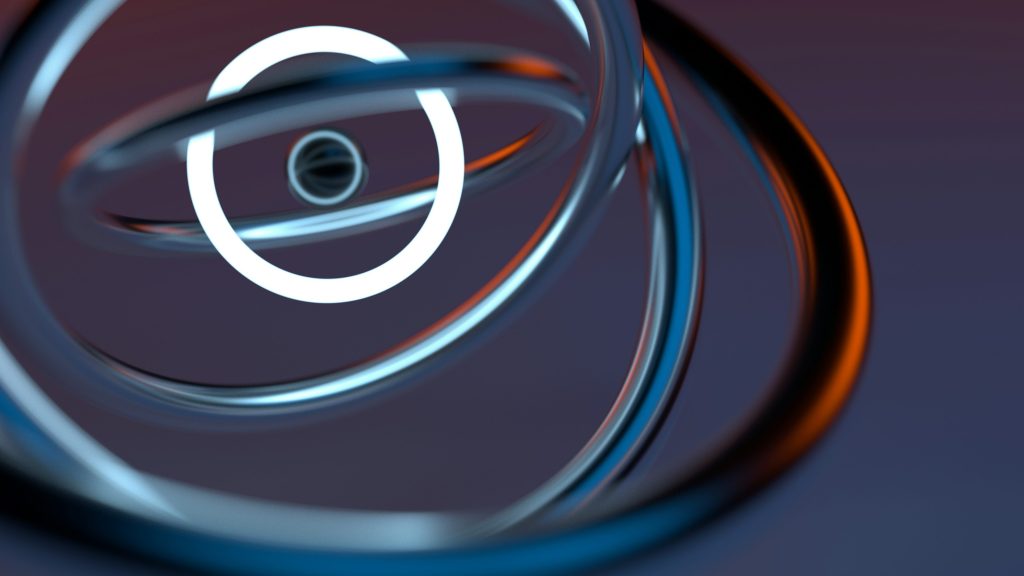
This article is published in collaboration with the AI Awards & Summit. Enter the awards by March 16, 2025 – click here to submit your entry. In this piece, Silicon Valley innovator Kevin Surace, known as the “Father of the Virtual Assistant,” explores the transformative power of AI and shares essential insights on its impact on businesses. In a world where AI is reshaping industries and redefining roles, my journey with AI has been guided by a commitment to solving real-world problems. From creating the first human-like virtual assistant, Portico, to developing Appvance’s AI-first platform for software quality, I’ve always believed in using AI to drive efficiency and innovation. Here, I’ll share some reflections on AI’s transformative impact, the challenges organisations face, and the future of technology in an increasingly AI-driven world. AI’s Impact on Business Processes and Decision-Making The advent of deep learning in 2012 marked a turning point. AI’s ability to perform complex computations enabled us to solve challenges we once thought impossible. Generative AI, the most publicised form of AI today, opened new possibilities, allowing anyone to interact with AI in natural language, making it accessible beyond traditional tech circles. In business, AI has revolutionised content creation and customer service. We can generate blog posts or complex imagery with a single prompt, driving the cost of content creation towards zero. This transformation extends to software development, where AI co-pilots can enhance productivity, making coding faster and more accurate. As I often say, AI is no longer a novelty; it’s a utility that boosts efficiency, speeds up processes, and redefines roles. Challenges in Implementing AI Technologies Despite AI’s potential, organisations face hurdles in adopting it. Privacy is paramount. When training models, companies must ensure proprietary data remains secure. Training is another challenge; without skilled AI trainers, many organisations struggle to make the most of AI tools. Cultural resistance also plays a role. Some employees may feel threatened by AI, viewing it as a replacement rather than a tool to enhance productivity. Overcoming these challenges requires a holistic approach. First, companies must secure and privatise their models to protect sensitive information. Training employees on AI usage is equally important to build comfort and proficiency with the technology. Lastly, leaders should emphasise AI as a means to enhance roles rather than replace them. A well-trained workforce empowered by AI is a recipe for sustained growth. Key Considerations for Long-Term Digital Transformation For organisations undergoing digital transformation, productivity gains should be the primary metric. Digital transformation isn’t just about adopting the latest tech—it’s about driving efficiency and cost-effectiveness. At Appvance, we harnessed AI to streamline software testing, eliminating repetitive tasks and allowing developers to focus on innovation. Our AIQ platform can generate and execute thousands of test cases automatically, providing full application coverage that was previously unachievable. This transformation requires organisations to set clear goals and identify areas where AI can deliver measurable results. Whether through improving customer service, enhancing employee satisfaction, or reducing operational costs, businesses should focus on AI as a tool for productivity and value creation. Ethical Considerations in AI Integration AI brings immense power, but it also raises ethical concerns. Privacy and security are crucial, particularly with technologies that handle sensitive data. Deepfakes and phishing scams are growing issues, with AI-generated messages nearly indistinguishable from human communication. To combat these threats, companies need robust cybersecurity measures. I’m working with Token Ring, a biometric ring that provides next-generation multi-factor authentication to secure applications against these risks. Bias in AI is another ethical issue. Models trained on historical data may reinforce stereotypes, creating unfair outcomes. For example, if an AI model consistently depicts CEOs as older white males, it limits representation for other demographics. To counteract this, companies need to audit their AI models for bias and ensure diverse training data to foster inclusivity. The Next Decade of AI: Exciting Developments and Industry Shifts Looking ahead, two trends stand out: the rise of AI agents and advances in humanoid robotics. AI agents, or autonomous digital assistants, will soon be capable of performing complex tasks on our behalf. Imagine telling an AI to schedule a meeting or negotiate a contract; these agents will revolutionise productivity by handling repetitive, administrative tasks. In robotics, reinforcement learning has enabled robots to perform intricate tasks without explicit programming. For instance, a robot can learn to make coffee by experimenting and “rewarding” successful attempts. This self-teaching capability opens up new possibilities for robots in everyday settings, from household chores to industrial applications. These developments are exciting because they bridge the digital and physical worlds. AI agents can handle digital tasks, while robots can assist in physical environments. Together, they will redefine what’s possible in industries from healthcare to manufacturing. Advice for Aspiring Innovators As someone who’s filed over 94 patents, I often reflect on my journey. My advice for young entrepreneurs is simple: focus on solving real problems. Innovation for its own sake is less impactful than finding solutions that meet a need or improve lives. Be resilient, learn from failures, and don’t be afraid to venture into diverse fields. Curiosity has driven my career, whether in AI, construction materials, or energy-efficient technologies. In today’s world, the pace of technological advancement is unprecedented. For those entering the field, remember that innovation is not just about having the best idea but about making it practical and valuable. Stay adaptable, focus on continuous learning, and surround yourself with people who challenge you. Conclusion AI is transforming every facet of business and life, from automating routine tasks to enabling unprecedented productivity gains. As AI continues to evolve, ethical considerations, privacy concerns, and workforce integration will remain crucial. For businesses and entrepreneurs, the key to leveraging AI lies in focusing on real-world applications, enhancing human capabilities, and maintaining a commitment to responsible innovation. By embracing AI thoughtfully, we can unlock new possibilities and build a future where technology serves humanity’s best interests. Get in touch For event sponsorship enquiries, please get in touch with calum.budge@31media.co.uk For media enquiries,
AI led Digital Transformation in Print Manufacturing
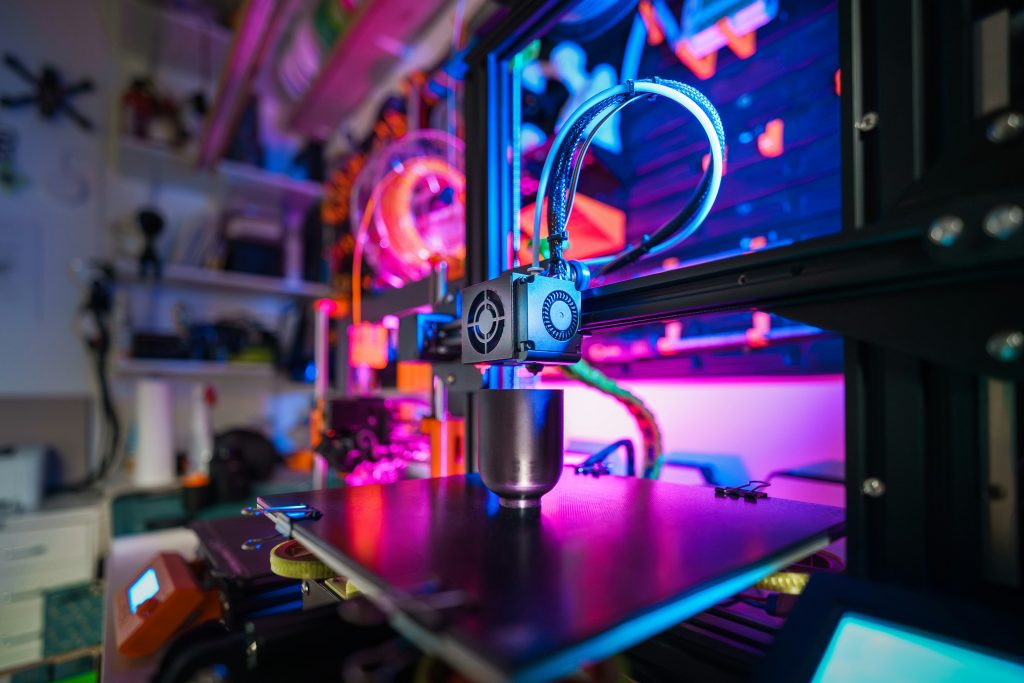
The manufacturing industry is undergoing a massive transformation, integrating technologies like artificial intelligence, automation, and digitalisation to optimise production and boost efficiency. In print manufacturing, companies adapt to these changes by viewing AI as a tool for growth rather than a threat. In this article, John Kilburg from K12 Printing reacts to digital transformation that is led by artificial intelligence. This article is published in line with the Digital Transformation and AI Awards and Summit. To exhibit at the event, please get in touch at +44 (0)203 931 5827. The Impact of Digital Transformation on Print Manufacturing This isn’t the first major shift in manufacturing. Like many other industries, print manufacturing experienced significant digital transformation with the introduction of computers and other digital technologies. Decades ago, manual processes dominated the industry, limiting efficiency and scope. The introduction of digital tools such as computer-aided design and automated printing presses revolutionised production. This shift allowed for faster workflows, improved precision, and greater customisation. This earlier wave of digital transformation was a cause of discomfort for many in the manufacturing industry due to concern about the new technology replacing jobs. Instead, it reshaped roles without replacing them. It created opportunities for workers to upskill and move into more specialised roles such as graphic designers or other important roles within the print manufacturing industry. Today, many print manufacturers see AI as a continuation of this evolution, offering new ways to enhance their business and empower their workforce. AI’s Role in Print Manufacturing While still early in print manufacturing, AI is poised to bring substantial benefits in certain areas. At K12 Print, AI is being explored cautiously, with a focus on enhancing efficiency and creativity rather than reducing jobs. Shortly, AI is likely to assist in design elements. This would enable designers to work faster and focus more on creativity and strategic decision-making. Another practical implementation is through machine maintenance, using predictive analytics to prevent breakdowns and optimise performance, minimising downtime. Rather than letting technology drive layoffs, companies should be committed to maintaining their workforce and creating opportunities for career advancement. This approach keeps jobs in the country and the community and strengthens the company’s foundation as a tight-knit group that values each employee’s growth. Enhancing Print Quality There are many practical uses of artificial intelligence in print manufacturing. First, AI can be used to analyse and optimise images that are received from the customer. Most customers are not knowledgeable about print files or colour builds. Artificial intelligence can sharpen images and adjust colour in real time. The result of these corrections is high-quality prints in a shorter amount of time which saves money. Also, AI may come into the design elements of print when customers bring forward ideas for us to create. Several nuances go into making their idea a reality and although there are currently real people building off those ideas. AI and Sustainability The manufacturing industry is constantly evolving when it comes to sustainability. Many of the printing tools have become more environmentally friendly over the years especially when it comes to ink and other critical elements in printing. But more changes on the horizon can make printing more sustainable. The use of AI systems can help reduce waste and save on energy consumption. This can help lessen the environmental impact of the printing industry. Additionally, artificial intelligence can use its vast database (learned knowledge) to suggest other areas where printing can transition to eco-friendly materials. A practical implementation of AI could also be in predictive maintenance—monitoring the machines in use and predicting breakdowns so they can be avoided. AI and Cost AI may be cost-prohibitive to smaller print manufacturing companies in the short term. However, as history teaches us, technology tends to become affordable very quickly once it is embraced by the masses. The more we learn to embrace AI rather than fear AI, the faster it will become a tool that propels us forward. Security Every data-driven industry must think about security. And print manufacturing is no different. Another practical implementation of AI in manufacturing is within the company’s security setup. AI can identify patterns in data traffic that can be indicative of a security breach and suggest, or even implement measures to counter the breach. It can also work to prevent security breaches by enforcing user authentication and even monitoring the printing of sensitive or confidential materials. Print Personalisation Although a vast majority of the print orders that come through the shop are custom orders there is still a major market for pre-made ready-to-order looks. AI systems can use available data about customers to customise content based on that data. This can be used in marketing campaigns to create high-response materials targeted to specific audiences. Packaging and direct mail will be big beneficiaries of this. AI Won’t Replace Jobs – It Will Create New Opportunities A common concern in the workplace is that AI will lead to job loss. However, this fear may be overstated. Much like the earlier digital transformation, AI presents an opportunity to elevate both workers and businesses. K12 Print is committed to investing in education and on-the-job training for employees, preparing them to adapt and thrive in a more technology-driven workplace. Upskilling the workforce will ensure that workers move into more rewarding roles. AI will likely take over repetitive tasks, but this shift will allow employees to focus on oversight roles. For print manufacturing, this means workers can explore new career paths in roles such as AI technicians, data analysts, and advanced machine operators. Digital transformation in manufacturing is inevitable, and AI will play a crucial role in shaping the future. Get in touch For event sponsorship enquiries, please get in touch with calum.budge@31media.co.uk For media enquiries, please get in touch with vaishnavi.nashte@31media.co.uk
Transforming Banking with DevOps
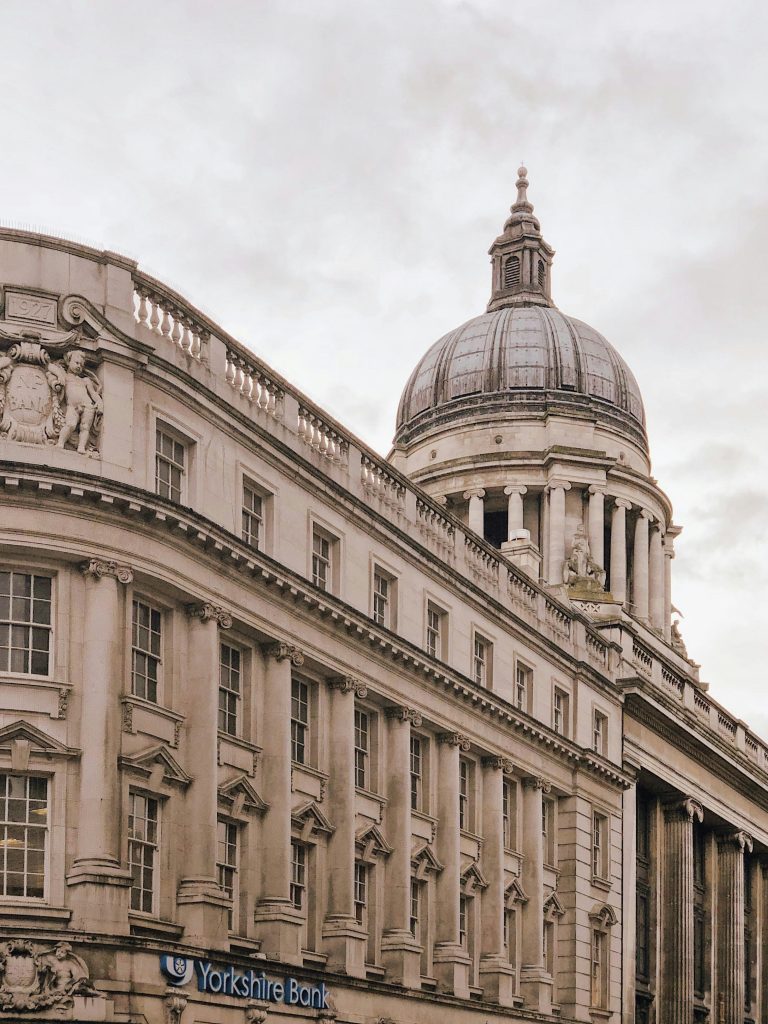
This article is published in collaboration with the Digital Transformation and AI Awards and Summit. These are two separate B2B events organised by 31 Media. If you wish to exhibit your tech solutions or to advertise your brand at the event, please get in touch at +44 (0)203 931 5827. Author: Arnab Mitra, programme manager at Banking Industry Architecture Network (BIAN) The banking industry is undergoing a significant digital transformation. The emergence of fintechs and industry disruptors is forcing traditional banks to innovate faster than ever before to remain competitive and address the needs of the digital-first customer. Delivering these solutions effectively and at speed often requires an overhaul of legacy technology and the emergence of new technological-driven processes. Enter DevOps, a combination of practices and tools that is driving the future of the financial services industry. We spoke with Arnab Mitra, programme manager at the Banking Industry Architecture Network (BIAN) about the role of DevOps in banking and the need for industry collaboration to further accelerate transformation. Q1: What challenges have traditionally hindered banks from adopting DevOps, and how has the industry’s perspective shifted to embrace this methodology? Traditionally, banks were slow to adopt DevOps due to regulatory constraints, concerns about data security, and a legacy mindset that is naturally more hesitant towards change and innovation. However, the pandemic accelerated the need for banks to offer digital services, which in turn hastened the adoption of DevOps. As the benefits of DevOps became clear, banks have realised that they needed to embrace this methodology to remain competitive and keep pace with nimbler fintechs, while offering new services that are enabled through best-of-breed technologies. In today’s rapidly evolving IT landscape, DevOps streamlines transformation and enables banks to deliver innovative digital services at speed and scale. With the global drive for transformation, banks recognised that if they don’t transform, they risk being left behind, and at a significant cost. For example, a 2023 IDC Financial Insights survey found global banks are on track to spend $57.1 billion on legacy payments technology in 2028. It’s therefore no surprise that over 80% of financial services firms have embraced DevOps practices, reflecting the widespread adoption and recognition of its benefits in the industry. Q2: What is BIAN’s approach to DevOps? How is this reflected in BIANs offerings? BIAN is built on collaboration across the industry and we use DevOps methodology within our member activities to encourage this. Working groups formed of members from different organisations within banks, technology vendors and consultancies come together to share thoughts, ideas and experiences to collaborate on innovative solutions, for example producing API specifications. Another example is our Coreless Banking initiative, which completed its third iteration last year. BIAN took a DevOps approach to this initiative, which was developed by a collaboration of leading banks and technology vendors, including HSBC, Zafin and IBM. The initiative, which aims to tackle the interoperability challenges banks face, resulted in an API-based services architecture that empowers banks to integrate best-of-breed technology seamlessly. Coreless Banking leverages the DevOps processes of the individual participating members to bring their components (solutions) into a published state, for other participants to use and integrate with. This allows for quick releases when any changes are required for any individual member. At the same time, using the BIAN standard for the API interface specifications means the integrated solution still works. Q3: Can you share any examples of how you’ve implemented DevOps principles on a more practical level? BIAN’s materials, including our Service Domains, are made available on the cloud, allowing members to access and use BIAN APIs for various applications within their organisations. Using BIAN’s framework, external parties can access our materials and create their own CI/CD pipeline, adapting it to their own needs. Members also have access to BIAN tooling, with functionality that allows users to match their APIs with BIAN APIs. In addition to this, we have an automated feedback loop and message modellers which enable rapid updates to BIAN models, once manually approved. Members can compare artefacts with the BIAN model content, helping to ensure APIs are compliant, within our framework. These automated processes guarantee consistent quality across all of our materials, eliminating individual preferences and ensuring regulatory compliance While we have been using DevOps in our approach for many years, we are now exploring how AI is enabling and evolving our DevOps operations. Q4: How is AI transforming DevOps practices and environments within the financial services industry? AI is a true game-changer within the industry. When applied to DevOps, the scope for automation within these environments is huge. BIAN is exploring many potential use cases for the technology. For instance, we’re looking at how we can use AI to generate sample data for Service Domain APIs to create a sandbox with quality test data that developers can use to mock up innovative solutions using these APIs. Additionally, we are piloting an AI-based API mapping app to automate the mapping process by 50-60%, significantly reducing human effort. We are focusing on training our AI-Engine with quality data, and feedback from our members supports the finetuning of this app. This means that members using our model will benefit from streamlined processes and enhanced efficiencies, while BIAN benefits from member feedback which continuously trains and improves our AI models, further supporting our collaborative environment. Q5: How important will DevOps be for the future of banking? Why is collaboration the key to transformation? As banks continue to focus on digital transformation, DevOps practices will be essential for delivering innovative products and services at speed. When development and operations teams work together closely, they can identify and address issues more efficiently, improve communication and ultimately deliver better results. By breaking down siloes – not only within organisations but across the financial services ecosystem – it creates a more cohesive and successful work environment. With the advent of new technologies, including AI, it’s now more important than ever for teams to share ideas about how this technology can be used safely and
Delivering DevOps through Platform Engineering
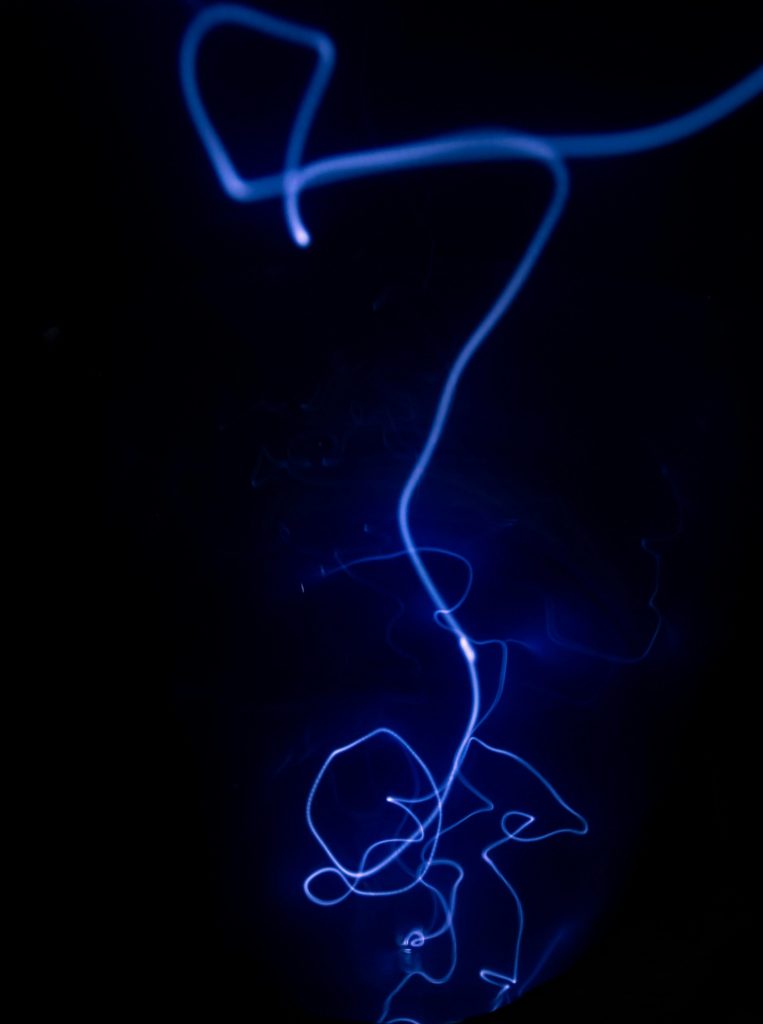
This article is published in collaboration with the Digital Transformation and AI Awards and Summit. These are two separate B2B events organised by 31 Media. If you wish to exhibit your tech solutions or to advertise your brand at the event, please get in touch at +44 (0)203 931 5827. Author: Fred Lherault, Field CTO, EMEA / Emerging Markets, Pure Storage Delivering on the promise of DevOps through Platform Engineering In software engineering, the Golden Path aims to provide the smoothest way forward via a self-service template for common tasks. It is enabled by platform engineers – who provide developers with the simplest possible internal developer platform and the tools they need to deliver innovation. Here we look at the emerging discipline of platform engineering, and the benefits it brings to application development via easier and faster access to services and resources, in particular using modern data management platforms built on Kubernetes containerised environments. Giving developers what they want When DevOps emerged in the late 2000s, it brought with it key principles of shared ownership, rapid feedback and workflow automation to help deliver on the vision of agile software development. It requires a high degree of autonomy for the developers and in exchange empowers them with the tools they need to be efficient. Automation is one of the key principles of DevOps since the quick pace of changes it drives is incompatible with “human in the loop” workflows. The mode of operations preferred by developers (and many technical specialist roles such as data scientists, AI researchers etc.) can often be boiled down to 3 main asks: Instant access to resources Instant results Full self-service Using the above as the “north star” when building services geared towards technical profiles is a great way to enable innovation and ensure fast adoption. While providing instant resources and results might not be always possible, getting as close as possible to instant will drive greater satisfaction. Platform engineering treats the developer as its primary customer Today, we see the coming of age of DevOps through the rise of platform engineering, a new function for a more mature era in application development, that provides a suite of self-service tools to empower developers. Platform engineering operates behind the veil to provide an easy-to-use, self-service catalogue of services and infrastructure components to support the day-to-day development experience. Best practice platform engineering aims to help application developers get on board and start building faster by providing everything that they need to experiment, develop, test and deploy. The platform made available to these developers often takes inspiration from the services popularised by the public cloud and its mode of operation. It is designed to provide instant access to not just the latest and greatest tools and software that underpin innovation, but also provide easy access to the data itself, protected by pre-determined guardrails and security protocols. Kubernetes and data management The ideal developer-focused platform also includes data management. It may build on top of Kubernetes as the means to orchestrate, deploy, run and scale cloud-native applications as well as to manage the data services required for those applications. Data management capabilities are key to platform engineering because they enable exploration and testing in realistic conditions, for example using an instant copy of production data instead of a somewhat unrealistic synthetic data set. Ideally, the data management capabilities will also be designed with self-service in mind, and deliver access to data in a highly available, reliable, elastic, multi-tenant and secure manner. Portworx from Pure Storage is an example of such a modern data platform. Fully integrated with Kubernetes, it allows the developer to easily get access to persistent data options (including data protection capabilities such as data replication, backup and archiving) but also to data sets themselves through instant data cloning, even enabling the use of self-service instant snapshot creation and restore so that developers may experiment with changes and roll back to previous states quickly and easily. Additionally, Portworx Data Services provides a catalogue of curated data services, including MongoDB, Elasticsearch, Cassandra, Kafka and PostgreSQL, simplifying deployment into just a few clicks or a single API call, so that developers can deploy or scale these data services easily with the optimal data storage configuration and protection. This foundation brings these easy-to-specify toolchains and data services to the developers so that they can easily use them as building blocks, even if they don’t have extensive knowledge of Kubernetes or how to deploy a given database engine in a secure and scalable manner. Platform engineering enables the Golden Path Platform engineering teams are busy working unseen in the background to bring the self-service Golden Path to application development. With Kubernetes as the orchestration framework, and containers and data services as key resources, the platform engineers can finally deliver fully on the vision of increased agility and greater productivity of DevOps. Get in touch For event sponsorship enquiries, please get in touch with calum.budge@31media.co.uk For media enquiries, please get in touch with vaishnavi.nashte@31media.co.uk
Using workflow automations to minimise development downtime
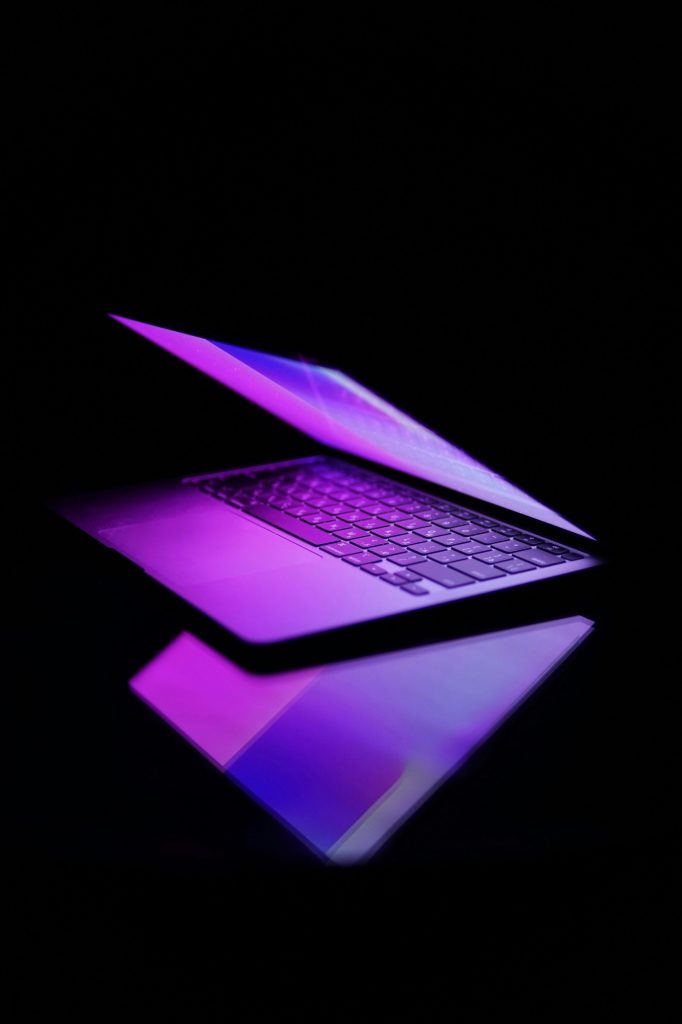
This article is published in collaboration with the Digital Transformation and AI Awards and Summit. These are two separate B2B events organised by 31 Media. If you wish to exhibit your tech solutions or to advertise your brand at the event, please get in touch at +44 (0)203 931 5827. In this article, you’ll learn how you can minimise development downtime with workflow automation. Author: Steve Barrett, VP of EMEA, Datadog Minimising development downtime and disruption through workflow automation DevOps teams are under constant pressure to deliver software applications as quickly as possible. At the same time, DevSecOps and security operations centre (SOC) teams face an ongoing challenge in detecting and remediating constantly evolving security threats. These challenges can be exacerbated by the various complex and often error-prone processes involved in responding to disruptions and changes to an organisation’s systems. A large amount of time can be spent switching between different tools to gather the context required for remediation, and in the manual execution of tasks needed for incident management, significantly prolonging downtime and causing further disruption. It can be hard to prioritise and manually respond to the high volumes of alerts generated by larger and more complex systems, further delaying resolution and increasing the risk of human error. Fortunately, a range of new workflow automation tools are available to support DevOps, DevSecOps and SOC teams, specifically in the observability and real-time monitoring of servers, databases, SaaS tools and services across their organisations’ cloud and IT infrastructure. Automate end-to-end processes Workflow automation helps teams more confidently manage the health of their systems and resolve issues faster, automating and orchestrating complex flows of tasks in response to specific threats, events, and alerts, and allowing teams to incorporate human input into those flows where required. By allowing them to combine monitoring and remediation into a single, streamlined solution, new workflow automation tools can enable DevOps to automate and orchestrate entire end-to-end processes across their infrastructure and tools, helping them to quickly remediate any issues that might arise. Consider alerts, for example. Whether monitoring network health, application performance, or infrastructure resources, DevOps teams must set alerts. By letting them know the moment an issue occurs, an alert allows them to respond in an appropriate and timely manner. But responding to alerts manually can be repetitive and time-consuming: an alert might send notifications in the middle of the night, or engineers might have to restart an application to resolve the issue manually. However, creating a workflow which consists of connected remedial actions that automatically execute when a specific alert is triggered can significantly reduce a team’s mean time to resolution (MTTR). Tackling emerging threats The technology has considerable benefits for DevSecOps and SOC teams, too, enabling them to orchestrate an automated series of actions in response to an alert, and quickly tackle any emerging threats to their system’s security. By chaining together specific actions in a workflow, or actions from integrations such as AWS, Okta and others, teams can configure workflows to trigger a specific alert and automatically execute an important security process, such as blocking a suspicious IP address, performing tier–one triage—such as reviewing and adding context to threats detected by cloud SIEM—or rolling back a code deployment that introduces a vulnerability. An organisation might use Okta for identity and access management, for example, and has a rule in place which detects when a user attempts to access an application without authorisation. Configuring and adding a “Suspend Suspicious Okta User” workflow means that if and when that rule is triggered, the suspicious user will be automatically suspended Workflow automation can even help create new rules that establish whether an alert has detected a real threat or a false positive. Although security signals provide much information, it’s not always enough to indicate whether an alert requires further investigation. By enriching cases with relevant context from the observability data generated through real-time monitoring, teams can better identify and eliminate false positives and determine whether an incident is a malicious event. DevSecOps and SOC teams can also combine new cloud SIEM and automated workflows to automate repetitive security tasks like detecting emerging vulnerabilities or triaging security signals. Traditionally separate automation, SIEM, and case management capabilities can be unified in a single pane of glass, allowing teams to create a centralised workspace for investigating their security signals. Not only does this help teams reduce tool sprawl and spending, but the combined use of cloud SIEM and automated workflows also reduces the burden on security engineers, allowing them to focus on more complex tasks. Streamlining monitoring and troubleshooting Today’s security teams operate in a constantly evolving, increasingly complex, and challenging environment. The use of disparate point solutions only adds to this complexity and can risk an ineffective security posture. Workflow automation helps mitigate this risk, streamlining monitoring and troubleshooting by automating end-to-end processes and executing actions in response to alerts, security threats, and other insights. As well as boosting productivity and saving valuable time, implementing automated workflows in response to security threats allows an organisation’s DevOps, DevSecOps, and SOC teams to focus on the most critical security issues and more quickly and easily detect and defend against potential attacks. Get in touch For event sponsorship enquiries, please get in touch with calum.budge@31media.co.uk For media enquiries, please get in touch with vaishnavi.nashte@31media.co.uk
Finalists announced for DevOps awards 2024
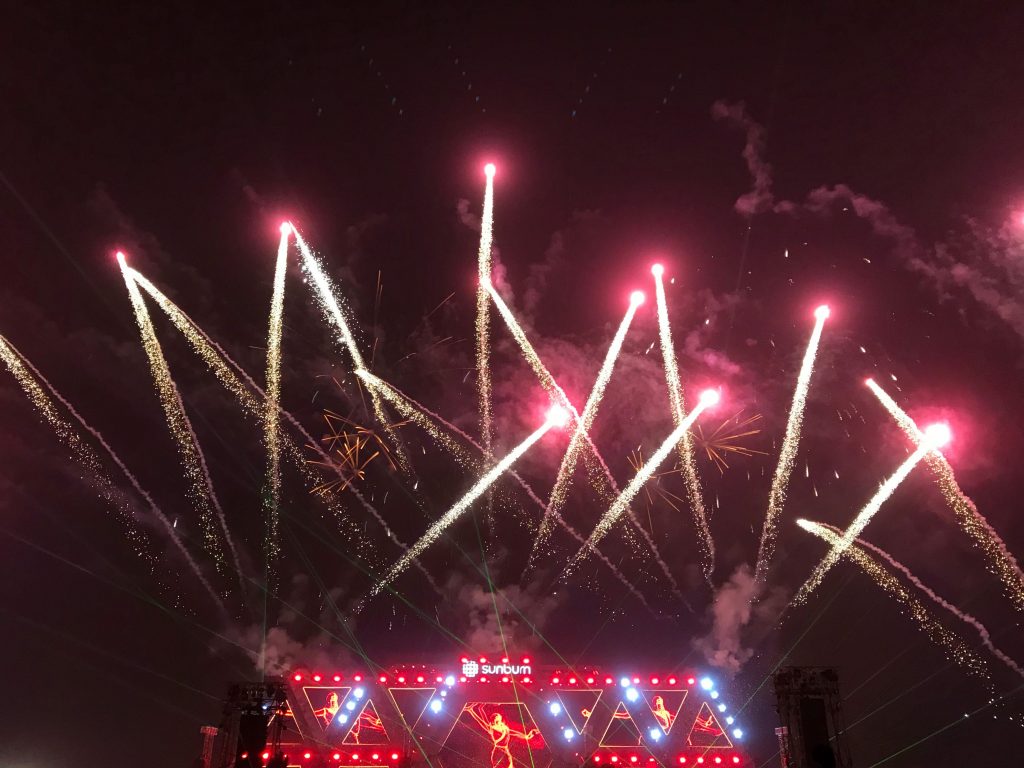
The finalists for the 2024 DevOps Awards were officially announced on September 30, 2024. Organised by 31 Media, this prestigious awards programme recognises excellence in DevOps across various industries, entering its 8th year of celebrating remarkable achievements. 31 Media has been at the forefront of organising industry-leading conferences and awards for over 17 years. The DevOps Awards are an independent, globally recognised programme, open to businesses, teams, and individuals across the world. With a multitude of categories, the programme allows multiple entries and provides a unique opportunity for participants to gain recognition for their contributions to the DevOps community. Reaching finalist status in these awards is a significant achievement, highlighting the talent, dedication, and innovative work of the competing companies and individuals. The event offers a chance to showcase your organisation’s expertise and elevate your brand visibility among top professionals in the DevOps and quality engineering sectors. The DevOps Awards ensure an impartial and transparent judging process. Judges, carefully selected for their extensive experience in the DevOps domain, hold senior leadership roles across diverse industries. To maintain complete fairness, all entries are judged anonymously, with identifying information removed. Winners are determined solely on merit, regardless of the size, budget, or influence of the company. Some of this year’s judges include: David Jambor – Senior Director, Tech and Secure Infrastructure at BCG Darren Griggs – CTO Advisory Matt Day – Head of App / Dev Technology Practice at Google Cloud UK/I Maria Stefanova – Head of Agile PMO and Digital Transformation at International Airlines Group Himanshu Kansal – Director of Engineering at Reed.co.uk Basit Tanveer – Head of Business Platforms at Lebara Chandri Krishnan – Engineering Leader at Meta Ravi Jay – Head of Agile Delivery at Jaguar Land Rover Lisa Li – Head of Engineering at Sainsbury’s Ruben Bell – Head of Technology Strategy & Governance Jason Ward – Head of Architecture & Engineering at Rethink Underwriting Ltd View the full list of finalists here. The 2024 DevOps Awards ceremony will take place in London on October 22-23, where the winners will be revealed. For table bookings or inquiries, please contact the team at grant@31media.co.uk.
How to build a digital-first company culture in recruitment
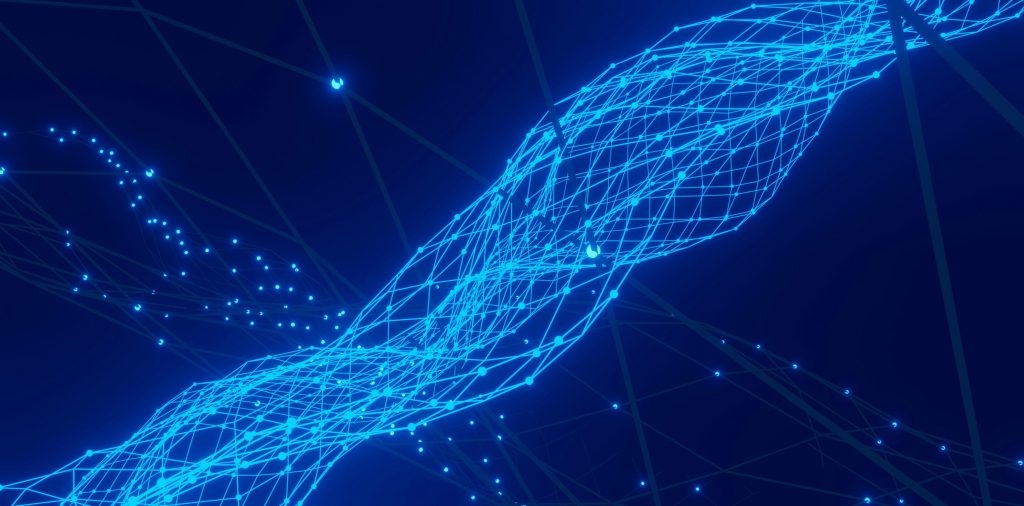
This article is published in collaboration with the Digital Transformation and AI Awards and Summit. These are two separate B2B events organised by 31 Media. If you wish to exhibit your tech solutions or to advertise your brand at the event, please get in touch at +44 (0)203 931 5827. In this article, you’ll learn how to build a digital-first company culture in talent acquisition Author: Gonzalo Guillen, CEO at HR Exchange Building a Digital-First Company Culture in Talent Acquisition As the world of work continues to evolve, companies are being challenged to rethink how they approach talent acquisition. The shift toward a digital-first strategy has transformed not only how we recruit but also how we build a company culture that thrives in a modern, tech-driven environment. I’ve had the privilege of working with companies across various industries, and I’ve seen firsthand how a digital-first approach can unlock new opportunities in talent acquisition. Here’s my perspective on what it takes to build a digital-first company culture in this critical area. The core principles of a digital-first company culture At the heart of a digital-first culture is the ability to harness technology to improve efficiency and scalability while keeping the human element intact. In talent acquisition, this means using digital tools like applicant tracking systems, AI-powered candidate sourcing, and communication platforms to enhance the recruitment process. But it’s not just about technology for technology’s sake—it’s about how you apply these tools to create a seamless experience for your team and the candidates. It’s essential to design processes that are efficient but also respectful of the candidate’s time and experience. Keeping the recruitment process personal in a digital world One of the biggest concerns with digital talent acquisition is the fear of losing the personal touch. We’ve all heard stories of candidates feeling like they’re just a number in the system. The truth is, that automation doesn’t have to be impersonal. With the right tools, you can create more personalised experiences. For example, AI-driven chatbots can handle initial inquiries quickly while tailoring responses based on candidate history. Video interviews and interactive assessments also allow us to get to know candidates better, ensuring that technology enhances – not replaces – the human connection. How Does Remote Work Enhance Collaboration in Talent Acquisition? Remote work has changed the game for recruiting teams. Tools like Slack, Zoom, and collaborative platforms allow teams to stay connected and move quickly, regardless of where they’re located. What I’ve found is that remote work, combined with the right digital tools, actually fosters better communication and collaboration. It creates a flexible environment where people can contribute meaningfully without being tied to a physical office. For talent acquisition, this means faster decisions, more diverse perspectives, and a stronger alignment with company culture. Assessing Soft Skills in a Digital-First Environment Soft skills are often harder to evaluate, especially when you’re not meeting candidates face-to-face. However, digital tools give us new ways to assess how someone thinks, communicates, and interacts with others. Video interviews are great for this – body language, tone, and communication style come through in ways that a resume can’t capture. Online simulations or real-world scenario tests are also excellent tools for evaluating a candidate’s problem-solving skills and adaptability. With these digital approaches, we can get a fuller picture of a candidate’s potential. Using Data to Shape a digital-first Talent Acquisition Strategy In a digital-first strategy, data is your best friend. When used correctly, data analytics can provide critical insights into your recruitment process, helping you identify areas for improvement and make smarter decisions. I’m a strong advocate for using metrics like time-to-hire, quality of hire, and candidate conversion rates to drive decisions. Predictive analytics can also help identify candidates who are more likely to thrive in your company’s environment, saving time and resources while increasing the chances of a successful hire. Real-World Examples of Successful Digital-First Talent Acquisition There are plenty of companies that have successfully implemented digital-first recruitment strategies, and I’ve had the pleasure of working with a few of them. Take IBM, for example – they’ve integrated AI throughout their hiring process, reducing the time to screen candidates while improving the quality of hires. They’ve also built a strong digital culture that supports remote work and collaboration. Another great example is Microsoft, where they’ve embraced digital tools to speed up recruitment and create an inclusive culture that prioritises employee well-being. The key to building a digital-first company culture in talent acquisition is striking the right balance between technology and human connection. When done correctly, it can transform the recruitment process, making it more efficient, data-driven, and candidate-focused. At the end of the day, it’s about leveraging digital tools to enhance – not replace – the relationships that make your company unique. Get in touch For event sponsorship enquiries, please get in touch with calum.budge@31media.co.uk For media enquiries, please get in touch with vaishnavi.nashte@31media.co.uk